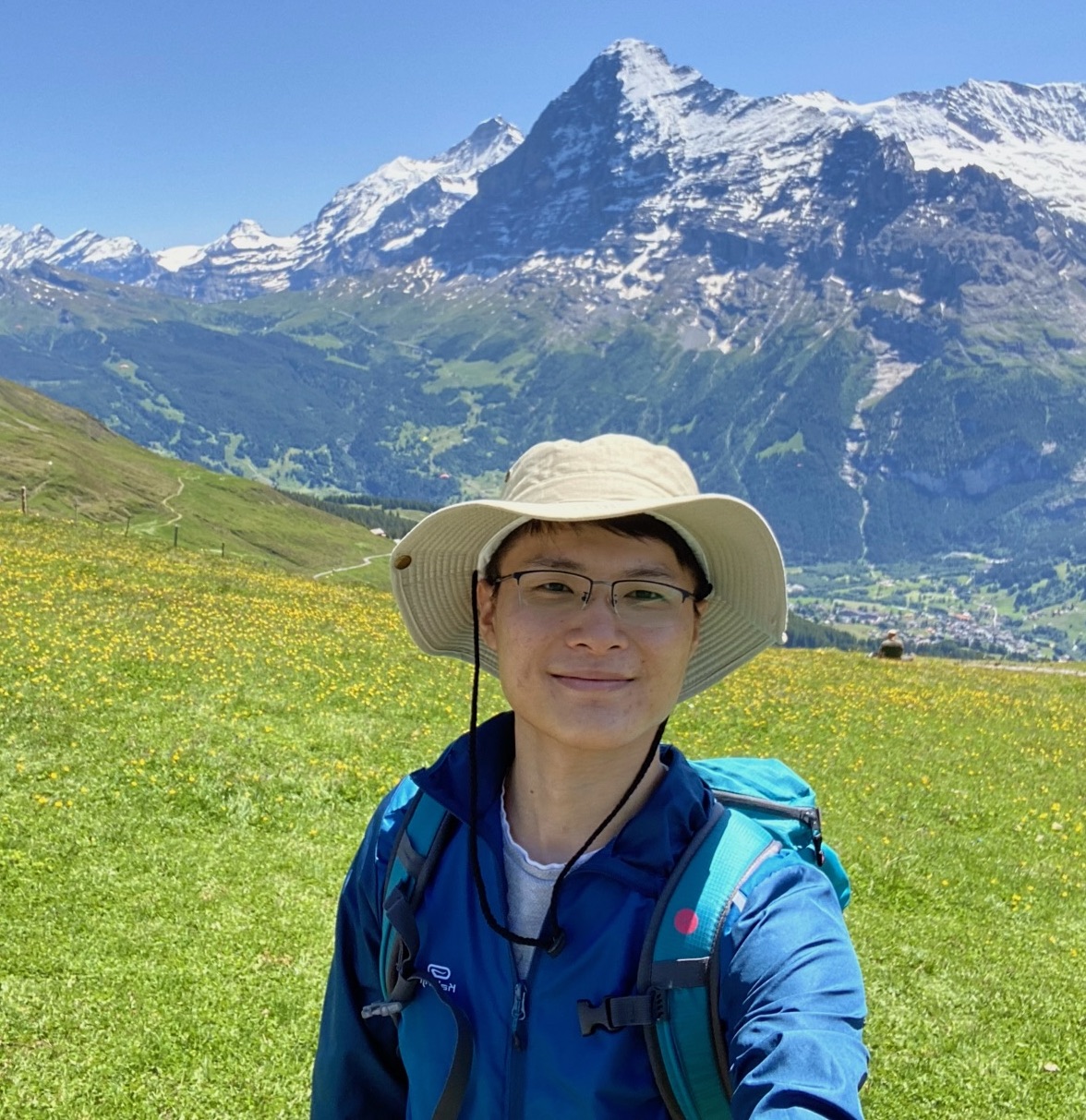
Shaohua Li
Assistant Professor
Department of Computer Science and Engineering
The Chinese University of Hong Kong
Email: shaohuali@cse.cuhk.edu.hk
Google Scholar | GitHub | Twitter
I am an Assistant Professor at the Department of Computer Science and Engineering, The Chinese University of Hong Kong. I am looking for PostDoc/Ph.D./RA/Intern students to join my group. If you’re interested, please drop me an email (shaohuali.cuhk@gmail.com) with your CV. See Openings for more details.
I recently obtained my Ph.D. at the AST Lab at ETH Zurich, advised by Zhendong Su. Previously, I got both my Master’s and Bachelor’s degrees from the University of Science and Technology of China (USTC).
News
- [Feb, 2025] Our SAND from ICSE 2025 has been upstreamed to AFL++ 🥳 6a4b580
- [Jan, 2025] Our paper on decoupling sanitization from fuzzing loop is accepted to ICSE 2025.
- [Oct, 2024] I will be serving as the PC member of PLDI 2025.
- [Jun, 2024] Our paper Creal receives the Distinguished Artifact Award at PLDI 2024.
- [May, 2024] I passed my Ph.D. defense 🥳. Prof. Mathias Payer and Prof. Andreas Zeller were on my committee.
- [Mar, 2024] Our paper is accepted to PLDI 2024.
- [Mar, 2024] I received the Student Travel Grant in ASPLOS 2024.
- [Jan, 2024] I was invited to join the PLDI 2024 Artifact Evaluation Committee.
- [Nov, 2023] Our paper UBFuzz received the Distinguished Artifact Award at ASPLOS 2024.
- [Oct, 2023] Our paper PGE received the Distinguished Paper Award at OOPSLA 2023.
Research Interests
I’m broadly interested in topics related to programming languages (PL), software engineering (SE) and security. In particular, I’m interested in the reliability and security of critical software systems such as compilers, databases, static/dynamic analysis tools, etc.
Well…finally, AI. I’m recently thinking about how to solve classic PL/SE/Security problems using AI. On the other hand, since AI models are still working as software/programs, they may also suffer from classic PL/SE/Security programs. Some of my recent research topics include not not limited to
- Compiler validation: Validating compilers’ behaviors via random testing or verification. Recently, I’m gaining more and more interests into the Rust compiler and deep learning compilers.
- Sanitization: Improve the reliability and efficiency of sanitization techniques, such as AddressSanitizer and MTE.
- Fuzzing: Detecting security vulnerabilities via dynamic testing.
- Deep learning systems: Reliability and efficiency of heterougeous DL systems such as TVM, PyTorch, Tensorflow, XLA, etc.
- LLM4Code: Functionality, security, and performance of LLM generated code.